Thinking in arrays#
Originally presented as part of HSF-India training on December 18, 2023.
So far, all the arrays we’ve dealt with have been rectangular (in $n$ dimensions; “rectilinear”).
What if we had data like this?
[
[[1.84, 0.324]],
[[-1.609, -0.713, 0.005], [0.953, -0.993, 0.011, 0.718]],
[[0.459, -1.517, 1.545], [0.33, 0.292]],
[[-0.376, -1.46, -0.206], [0.65, 1.278]],
[[], [], [1.617]],
[]
]
[
[[-0.106, 0.611]],
[[0.118, -1.788, 0.794, 0.658], [-0.105]]
]
[
[[-0.384], [0.697, -0.856]],
[[0.778, 0.023, -1.455, -2.289], [-0.67], [1.153, -1.669, 0.305, 1.517, -0.292]]
]
[
[[0.205, -0.355], [-0.265], [1.042]],
[[-0.004], [-1.167, -0.054, 0.726, 0.213]],
[[1.741, -0.199, 0.827]]
]
What if we had data like this?
[
{"fill": "#b1b1b1", "stroke": "none", "points": [{"x": 5.27453, "y": 1.03276},
{"x": -3.51280, "y": 1.74849}]},
{"fill": "#b1b1b1", "stroke": "none", "points": [{"x": 8.21630, "y": 4.07844},
{"x": -0.79157, "y": 3.49478}, {"x": 16.38932, "y": 5.29399},
{"x": 10.38641, "y": 0.10832}, {"x": -2.07070, "y": 14.07140},
{"x": 9.57021, "y": -0.94823}, {"x": 1.97332, "y": 3.62380},
{"x": 5.66760, "y": 11.38001}, {"x": 0.25497, "y": 3.39276},
{"x": 3.86585, "y": 6.22051}, {"x": -0.67393, "y": 2.20572}]},
{"fill": "#d0d0ff", "stroke": "none", "points": [{"x": 3.59528, "y": 7.37191},
{"x": 0.59192, "y": 2.91503}, {"x": 4.02932, "y": -1.13601},
{"x": -1.01593, "y": 1.95894}, {"x": 1.03666, "y": 0.05251}]},
{"fill": "#d0d0ff", "stroke": "none", "points": [{"x": -8.78510, "y": -0.00497},
{"x": -15.22688, "y": 3.90244}, {"x": 5.74593, "y": 4.12718}]},
{"fill": "none", "stroke": "#000000", "points": [{"x": 4.40625, "y": -6.953125},
{"x": 4.34375, "y": -7.09375}, {"x": 4.3125, "y": -7.140625},
{"x": 4.140625, "y": -7.140625}]},
{"fill": "none", "stroke": "#808080", "points": [{"x": 0.46875, "y": -0.09375},
{"x": 0.46875, "y": -0.078125}, {"x": 0.46875, "y": 0.53125}]}
]
What if we had data like this?
[
{"movie": "Evil Dead", "year": 1981, "actors":
["Bruce Campbell", "Ellen Sandweiss", "Richard DeManincor", "Betsy Baker"]
},
{"movie": "Darkman", "year": 1900, "actors":
["Liam Neeson", "Frances McDormand", "Larry Drake", "Bruce Campbell"]
},
{"movie": "Army of Darkness", "year": 1992, "actors":
["Bruce Campbell", "Embeth Davidtz", "Marcus Gilbert", "Bridget Fonda",
"Ted Raimi", "Patricia Tallman"]
},
{"movie": "A Simple Plan", "year": 1998, "actors":
["Bill Paxton", "Billy Bob Thornton", "Bridget Fonda", "Brent Briscoe"]
},
{"movie": "Spider-Man 2", "year": 2004, "actors":
["Tobey Maguire", "Kristen Dunst", "Alfred Molina", "James Franco",
"Rosemary Harris", "J.K. Simmons", "Stan Lee", "Bruce Campbell"]
},
{"movie": "Drag Me to Hell", "year": 2009, "actors":
["Alison Lohman", "Justin Long", "Lorna Raver", "Dileep Rao", "David Paymer"]
}
]
What if we had data like this?
[
{"run": 1, "luminosityBlock": 156, "event": 46501,
"PV": {"x": 0.243, "y": 0.393, "z": 1.451},
"electron": [],
"muon": [
{"pt": 63.043, "eta": -0.718, "phi": 2.968, "mass": 0.105, "charge": 1},
{"pt": 38.120, "eta": -0.879, "phi": -1.032, "mass": 0.105, "charge": -1},
{"pt": 4.048, "eta": -0.320, "phi": 1.038, "mass": 0.105, "charge": 1}
],
"MET": {"pt": 21.929, "phi": -2.730}
},
{"run": 1, "luminosityBlock": 156, "event": 46502,
"PV": {"x": 0.244, "y": 0.395, "z": -2.879},
"electron": [
{"pt": 21.902, "eta": -0.702, "phi": 0.133, "mass": 0.005, "charge": 1},
{"pt": 42.632, "eta": -0.979, "phi": -1.863, "mass": 0.008, "charge": 1},
{"pt": 78.012, "eta": -0.933, "phi": -2.207, "mass": 0.018, "charge": -1},
{"pt": 23.835, "eta": -1.362, "phi": -0.621, "mass": 0.008, "charge": -1}
],
"muon": [],
"MET": {"pt": 16.972, "phi": 2.866}},
...
]
It might be possible to turn these datasets into tabular form using surrogate keys and database normalization, but
they could be inconvenient or less efficient in that form, depending on what we want to do,
they were very likely given in a ragged/untidy form. You can’t ignore the data-cleaning step!
Dealing with these datasets as JSON or Python objects is inefficient for the same reason as for lists of numbers.
We want arbitrary data structure with array-oriented interface and performance…
Libraries for irregular arrays#
import pyarrow as pa
arrow_array = pa.array([
[{"x": 1.1, "y": [1]}, {"x": 2.2, "y": [1, 2]}, {"x": 3.3, "y": [1, 2, 3]}],
[],
[{"x": 4.4, "y": [1, 2, 3, 4]}, {"x": 5.5, "y": [1, 2, 3, 4, 5]}]
])
arrow_array.type
ListType(list<item: struct<x: double, y: list<item: int64>>>)
arrow_array
<pyarrow.lib.ListArray object at 0x7f6034942740>
[
-- is_valid: all not null
-- child 0 type: double
[
1.1,
2.2,
3.3
]
-- child 1 type: list<item: int64>
[
[
1
],
[
1,
2
],
[
1,
2,
3
]
],
-- is_valid: all not null
-- child 0 type: double
[]
-- child 1 type: list<item: int64>
[],
-- is_valid: all not null
-- child 0 type: double
[
4.4,
5.5
]
-- child 1 type: list<item: int64>
[
[
1,
2,
3,
4
],
[
1,
2,
3,
4,
5
]
]
]
import awkward as ak
awkward_array = ak.from_arrow(arrow_array)
awkward_array
[[{x: 1.1, y: [1]}, {x: 2.2, y: [...]}, {x: 3.3, y: [1, 2, 3]}], [], [{x: 4.4, y: [1, 2, 3, 4]}, {x: 5.5, y: [1, ..., 5]}]] ---------------------------------------------------------------- type: 3 * var * ?{ x: ?float64, y: option[var * ?int64] }
ak.to_parquet(awkward_array, "/tmp/file.parquet")
<pyarrow._parquet.FileMetaData object at 0x7f6034152de0>
created_by: parquet-cpp-arrow version 16.0.0
num_columns: 2
num_rows: 3
num_row_groups: 1
format_version: 2.6
serialized_size: 0
ak.from_parquet("/tmp/file.parquet")
[[{x: 1.1, y: [1]}, {x: 2.2, y: [...]}, {x: 3.3, y: [1, 2, 3]}], [], [{x: 4.4, y: [1, 2, 3, 4]}, {x: 5.5, y: [1, ..., 5]}]] ---------------------------------------------------------------- type: 3 * var * ?{ x: ?float64, y: option[var * ?int64] }
Awkward Array#
ragged = ak.Array([
[
[[1.84, 0.324]],
[[-1.609, -0.713, 0.005], [0.953, -0.993, 0.011, 0.718]],
[[0.459, -1.517, 1.545], [0.33, 0.292]],
[[-0.376, -1.46, -0.206], [0.65, 1.278]],
[[], [], [1.617]],
[]
],
[
[[-0.106, 0.611]],
[[0.118, -1.788, 0.794, 0.658], [-0.105]]
],
[
[[-0.384], [0.697, -0.856]],
[[0.778, 0.023, -1.455, -2.289], [-0.67], [1.153, -1.669, 0.305, 1.517, -0.292]]
],
[
[[0.205, -0.355], [-0.265], [1.042]],
[[-0.004], [-1.167, -0.054, 0.726, 0.213]],
[[1.741, -0.199, 0.827]]
]
])
Multidimensional indexing
ragged[3, 1, -1, 2]
0.726
Basic slicing
ragged[3, 1:, -1, 1:3]
[[-0.054, 0.726], [-0.199, 0.827]] ----------------------- type: 2 * var * float64
Advanced slicing
ragged[[False, False, True, True], [0, -1, 0, -1], 0, -1]
[-0.384, 0.827] ----------------- type: 2 * float64
Awkward slicing
ragged > 0
[[[[True, True]], [[False, False, True], [True, ..., True]], ..., [...], []], [[[False, True]], [[True, False, True, True], [False]]], [[[False], [True, False]], [[True, True, False, False], ..., [...]]], [[[True, False], [False], [True]], [[False], ...], [[True, False, True]]]] ----------------------------------------------------------------------------- type: 4 * var * var * var * bool
ragged[ragged > 0]
[[[[1.84, 0.324]], [[0.005], [0.953, 0.011, 0.718]], [...], ..., [[], ...], []], [[[0.611]], [[0.118, 0.794, 0.658], []]], [[[], [0.697]], [[0.778, 0.023], [], [1.15, 0.305, 1.52]]], [[[0.205], [], [1.04]], [[], [0.726, 0.213]], [[1.74, 0.827]]]] -------------------------------------------------------------------------------- type: 4 * var * var * var * float64
Reductions
ak.sum(ragged)
2.8980000000000006
ak.sum(ragged, axis=-1)
[[[2.16], [-2.32, 0.689], [0.487, 0.622], [-2.04, ...], [0, 0, 1.62], []], [[0.505], [-0.218, -0.105]], [[-0.384, -0.159], [-2.94, -0.67, 1.01]], [[-0.15, -0.265, 1.04], [-0.004, -0.282], [2.37]]] -------------------------------------------------------------------------- type: 4 * var * var * float64
ak.sum(ragged, axis=0)
[[[1.56, 0.58], [0.432, -0.856], [1.04]], [[-0.717, -2.48, -0.656, -1.63], ..., [1.15, -1.67, 0.305, 1.52, -0.292]], [[2.2, -1.72, 2.37], [0.33, 0.292]], [[-0.376, -1.46, -0.206], [0.65, 1.28]], [[], [], [1.62]], []] --------------------------------------------------------------------------- type: 6 * var * var * float64
How do we even define reductions on an array with variable length lists?
How do we even define reductions on an array with variable length lists?
array = ak.Array([[ 1, 2, 3, 4],
[ 10, None, 30 ],
[ 100, 200 ]])
ak.sum(array, axis=0).tolist()
[111, 202, 33, 4]
ak.sum(array, axis=1).tolist()
[10, 40, 300]
(You almost always want the deepest/maximum axis
, which you can get with axis=-1
.)
Awkward Arrays in particle physics#
import uproot
file = uproot.open("https://github.com/jpivarski-talks/2023-12-18-hsf-india-tutorial-bhubaneswar/raw/main/data/SMHiggsToZZTo4L.root")
file
<ReadOnlyDirectory '/' at 0x7f6058409110>
tree = file["Events"]
tree
<TTree 'Events' (32 branches) at 0x7f6030f092d0>
tree.arrays(entry_stop=100)
[{run: 1, luminosityBlock: 156, event: 46501, PV_npvs: 15, PV_x: 0.244, ...}, {run: 1, luminosityBlock: 156, event: 46502, PV_npvs: 13, PV_x: 0.244, ...}, {run: 1, luminosityBlock: 156, event: 46503, PV_npvs: 11, PV_x: 0.242, ...}, {run: 1, luminosityBlock: 156, event: 46504, PV_npvs: 22, PV_x: 0.243, ...}, {run: 1, luminosityBlock: 156, event: 46505, PV_npvs: 18, PV_x: 0.24, ...}, {run: 1, luminosityBlock: 156, event: 46506, PV_npvs: 5, PV_x: 0.243, ...}, {run: 1, luminosityBlock: 156, event: 46507, PV_npvs: 11, PV_x: 0.242, ...}, {run: 1, luminosityBlock: 156, event: 46508, PV_npvs: 25, PV_x: 0.245, ...}, {run: 1, luminosityBlock: 156, event: 46509, PV_npvs: 12, PV_x: 0.246, ...}, {run: 1, luminosityBlock: 156, event: 46510, PV_npvs: 18, PV_x: 0.244, ...}, ..., {run: 1, luminosityBlock: 156, event: 46592, PV_npvs: 26, PV_x: 0.243, ...}, {run: 1, luminosityBlock: 156, event: 46593, PV_npvs: 12, PV_x: 0.243, ...}, {run: 1, luminosityBlock: 156, event: 46594, PV_npvs: 8, PV_x: 0.246, ...}, {run: 1, luminosityBlock: 156, event: 46595, PV_npvs: 17, PV_x: 0.243, ...}, {run: 1, luminosityBlock: 156, event: 46596, PV_npvs: 17, PV_x: 0.245, ...}, {run: 1, luminosityBlock: 156, event: 46597, PV_npvs: 14, PV_x: 0.243, ...}, {run: 1, luminosityBlock: 156, event: 46598, PV_npvs: 22, PV_x: 0.243, ...}, {run: 1, luminosityBlock: 156, event: 46599, PV_npvs: 13, PV_x: 0.245, ...}, {run: 1, luminosityBlock: 156, event: 46600, PV_npvs: 21, PV_x: 0.246, ...}] ----------------------------------------------------------------------------- type: 100 * { run: int32, luminosityBlock: uint32, event: uint64, PV_npvs: int32, PV_x: float32, PV_y: float32, PV_z: float32, nMuon: uint32, Muon_pt: var * float32, Muon_eta: var * float32, Muon_phi: var * float32, Muon_mass: var * float32, Muon_charge: var * int32, Muon_pfRelIso03_all: var * float32, Muon_pfRelIso04_all: var * float32, Muon_dxy: var * float32, Muon_dxyErr: var * float32, Muon_dz: var * float32, Muon_dzErr: var * float32, nElectron: uint32, Electron_pt: var * float32, Electron_eta: var * float32, Electron_phi: var * float32, Electron_mass: var * float32, Electron_charge: var * int32, Electron_pfRelIso03_all: var * float32, Electron_dxy: var * float32, Electron_dxyErr: var * float32, Electron_dz: var * float32, Electron_dzErr: var * float32, MET_pt: float32, MET_phi: float32 }
The same data fits into Parquet files (a little more easily).
events = ak.from_parquet("https://github.com/jpivarski-talks/2023-12-18-hsf-india-tutorial-bhubaneswar/raw/main/data/SMHiggsToZZTo4L.parquet")
events
[{run: 1, luminosityBlock: 156, event: 46501, PV: {...}, electron: [], ...}, {run: 1, luminosityBlock: 156, event: 46502, PV: {...}, electron: [...], ...}, {run: 1, luminosityBlock: 156, event: 46503, PV: {...}, electron: [...], ...}, {run: 1, luminosityBlock: 156, event: 46504, PV: {...}, electron: ..., ...}, {run: 1, luminosityBlock: 156, event: 46505, PV: {...}, electron: [...], ...}, {run: 1, luminosityBlock: 156, event: 46506, PV: {...}, electron: ..., ...}, {run: 1, luminosityBlock: 156, event: 46507, PV: {...}, electron: ..., ...}, {run: 1, luminosityBlock: 156, event: 46508, PV: {...}, electron: ..., ...}, {run: 1, luminosityBlock: 156, event: 46509, PV: {...}, electron: [...], ...}, {run: 1, luminosityBlock: 156, event: 46510, PV: {...}, electron: [], ...}, ..., {run: 1, luminosityBlock: 996, event: 298792, PV: {...}, electron: [...], ...}, {run: 1, luminosityBlock: 996, event: 298793, PV: {...}, electron: ..., ...}, {run: 1, luminosityBlock: 996, event: 298794, PV: {...}, electron: [], ...}, {run: 1, luminosityBlock: 996, event: 298795, PV: {...}, electron: [...], ...}, {run: 1, luminosityBlock: 996, event: 298796, PV: {...}, electron: [], ...}, {run: 1, luminosityBlock: 996, event: 298797, PV: {...}, electron: ..., ...}, {run: 1, luminosityBlock: 996, event: 298798, PV: {...}, electron: [], ...}, {run: 1, luminosityBlock: 996, event: 298799, PV: {...}, electron: [...], ...}, {run: 1, luminosityBlock: 996, event: 298800, PV: {...}, electron: [...], ...}] -------------------------------------------------------------------------------- type: 299973 * { run: int32, luminosityBlock: int64, event: uint64, PV: Vector3D[ x: float32, y: float32, z: float32 ], electron: var * Momentum4D[ pt: float32, eta: float32, phi: float32, mass: float32, charge: int32, pfRelIso03_all: float32, dxy: float32, dxyErr: float32, dz: float32, dzErr: float32 ], muon: var * Momentum4D[ pt: float32, eta: float32, phi: float32, mass: float32, charge: int32, pfRelIso03_all: float32, pfRelIso04_all: float32, dxy: float32, dxyErr: float32, dz: float32, dzErr: float32 ], MET: Momentum2D[ pt: float32, phi: float32 ] }
View the first event as Python lists and dicts (like JSON).
events[0].to_list()
{'run': 1,
'luminosityBlock': 156,
'event': 46501,
'PV': {'x': 0.24369880557060242,
'y': 0.3936990201473236,
'z': 1.451307773590088},
'electron': [],
'muon': [{'pt': 63.04386901855469,
'eta': -0.7186822295188904,
'phi': 2.968005895614624,
'mass': 0.10565836727619171,
'charge': 1,
'pfRelIso03_all': 0.0,
'pfRelIso04_all': 0.0,
'dxy': -0.004785160068422556,
'dxyErr': 0.0060764215886592865,
'dz': 0.09005985409021378,
'dzErr': 0.044572051614522934},
{'pt': 38.12034606933594,
'eta': -0.8794569969177246,
'phi': -1.0324749946594238,
'mass': 0.10565836727619171,
'charge': -1,
'pfRelIso03_all': 0.0,
'pfRelIso04_all': 0.0,
'dxy': 0.0005746808601543307,
'dxyErr': 0.0013040687190368772,
'dz': -0.0032290113158524036,
'dzErr': 0.003023269586265087},
{'pt': 4.04868745803833,
'eta': -0.320764422416687,
'phi': 1.0385035276412964,
'mass': 0.10565836727619171,
'charge': 1,
'pfRelIso03_all': 0.0,
'pfRelIso04_all': 0.17997965216636658,
'dxy': -0.00232272082939744,
'dxyErr': 0.004343290813267231,
'dz': -0.005162843037396669,
'dzErr': 0.004190043080598116}],
'MET': {'pt': 21.929929733276367, 'phi': -2.7301223278045654}}
Get one numeric field (also known as “column”).
events.electron.pt
[[], [21.9, 42.6, 78, 23.8], [11.6, 6.69], [10.4], [30.7, 29.2, 6.38, 6.24], [16.1], [5.32], [6.99], [41, 6.5, 13.1, 52.1], [], ..., [19.1, 9.69], [30.2], [], [37, 7.36, 48], [], [12.3], [], [17.9, 23.2], [48.1, 38.7]] ---------------------------- type: 299973 * var * float32
Compute something ($p_z = p_T \sinh\eta$).
import numpy as np
events.electron.pt * np.sinh(events.electron.eta)
[[], [-16.7, -48.8, -83.9, -43.5], [-11.1, 26], [-0.237], [-19.9, 47.5, -18, -15.1], [5.58], [-3.22], [3.88], [-8.92, -10.5, -23.8, -17.3], [], ..., [26.4, 19.1], [92.2], [], [-193, -2.78, -43.4], [], [-3.4], [], [80.1, 99.3], [26.8, 74]] ------------------------------ type: 299973 * var * float32
Note that the Vector library works with Awkward Arrays, if it is imported this way:
import vector
vector.register_awkward()
Records with name="Momentum4D"
and fields with coordinate names (px
, py
, pz
, E
or pt
, phi
, eta
, m
) automatically get Vector properties and methods.
events.electron.type.show()
299973 * var * Momentum4D[
pt: float32,
eta: float32,
phi: float32,
mass: float32,
charge: int32,
pfRelIso03_all: float32,
dxy: float32,
dxyErr: float32,
dz: float32,
dzErr: float32
]
# implicitly computes pz = pt * sinh(eta)
events.electron.pz
[[], [-16.7, -48.8, -83.9, -43.5], [-11.1, 26], [-0.237], [-19.9, 47.5, -18, -15.1], [5.58], [-3.22], [3.88], [-8.92, -10.5, -23.8, -17.3], [], ..., [26.4, 19.1], [92.2], [], [-193, -2.78, -43.4], [], [-3.4], [], [80.1, 99.3], [26.8, 74]] ------------------------------ type: 299973 * var * float32
To make histograms or other plots, we need numbers without structure, so ak.flatten()
the array.
from hist import Hist
Hist.new.Regular(100, 0, 100, name=" ").Double().fill(
ak.flatten(events.electron.pt)
).plot();
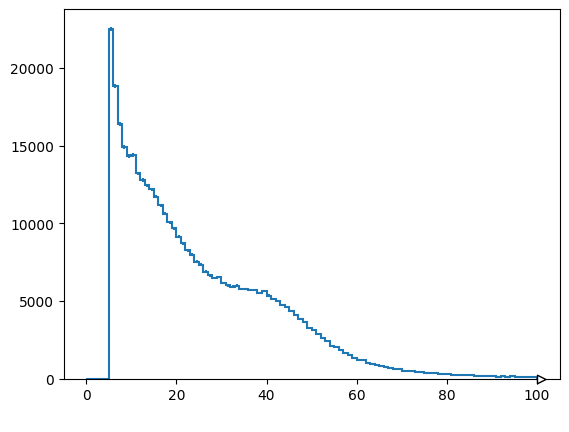
Each event has a different number of electrons and muons (ak.num()
to check).
ak.num(events.electron), ak.num(events.muon)
(<Array [0, 4, 2, 1, 4, 1, 1, 1, ..., 0, 3, 0, 1, 0, 2, 2] type='299973 * int64'>,
<Array [3, 0, 0, 7, 0, 2, 1, 0, ..., 2, 0, 2, 2, 4, 0, 0] type='299973 * int64'>)
So what happens if we try to compute something with the electrons’ $p_T$ and the muons’ $\eta$?
events.electron.pt * np.sinh(events.muon.eta)
---------------------------------------------------------------------------
ValueError Traceback (most recent call last)
Cell In[33], line 1
----> 1 events.electron.pt * np.sinh(events.muon.eta)
File ~/micromamba/envs/awkward-docs/lib/python3.11/site-packages/awkward/_operators.py:53, in _binary_method.<locals>.func(self, other)
51 if _disables_array_ufunc(other):
52 return NotImplemented
---> 53 return ufunc(self, other)
File ~/micromamba/envs/awkward-docs/lib/python3.11/site-packages/awkward/highlevel.py:1511, in Array.__array_ufunc__(self, ufunc, method, *inputs, **kwargs)
1509 name = f"{type(ufunc).__module__}.{ufunc.__name__}.{method!s}"
1510 with ak._errors.OperationErrorContext(name, inputs, kwargs):
-> 1511 return ak._connect.numpy.array_ufunc(ufunc, method, inputs, kwargs)
File ~/micromamba/envs/awkward-docs/lib/python3.11/site-packages/awkward/_connect/numpy.py:466, in array_ufunc(ufunc, method, inputs, kwargs)
458 raise TypeError(
459 "no {}.{} overloads for custom types: {}".format(
460 type(ufunc).__module__, ufunc.__name__, ", ".join(error_message)
461 )
462 )
464 return None
--> 466 out = ak._broadcasting.broadcast_and_apply(
467 inputs, action, allow_records=False, function_name=ufunc.__name__
468 )
470 if len(out) == 1:
471 return wrap_layout(out[0], behavior=behavior, attrs=attrs)
File ~/micromamba/envs/awkward-docs/lib/python3.11/site-packages/awkward/_broadcasting.py:1108, in broadcast_and_apply(inputs, action, depth_context, lateral_context, allow_records, left_broadcast, right_broadcast, numpy_to_regular, regular_to_jagged, function_name, broadcast_parameters_rule)
1106 backend = backend_of(*inputs, coerce_to_common=False)
1107 isscalar = []
-> 1108 out = apply_step(
1109 backend,
1110 broadcast_pack(inputs, isscalar),
1111 action,
1112 0,
1113 depth_context,
1114 lateral_context,
1115 {
1116 "allow_records": allow_records,
1117 "left_broadcast": left_broadcast,
1118 "right_broadcast": right_broadcast,
1119 "numpy_to_regular": numpy_to_regular,
1120 "regular_to_jagged": regular_to_jagged,
1121 "function_name": function_name,
1122 "broadcast_parameters_rule": broadcast_parameters_rule,
1123 },
1124 )
1125 assert isinstance(out, tuple)
1126 return tuple(broadcast_unpack(x, isscalar) for x in out)
File ~/micromamba/envs/awkward-docs/lib/python3.11/site-packages/awkward/_broadcasting.py:1086, in apply_step(backend, inputs, action, depth, depth_context, lateral_context, options)
1084 return result
1085 elif result is None:
-> 1086 return continuation()
1087 else:
1088 raise AssertionError(result)
File ~/micromamba/envs/awkward-docs/lib/python3.11/site-packages/awkward/_broadcasting.py:1055, in apply_step.<locals>.continuation()
1053 # Any non-string list-types?
1054 elif any(x.is_list and not is_string_like(x) for x in contents):
-> 1055 return broadcast_any_list()
1057 # Any RecordArrays?
1058 elif any(x.is_record for x in contents):
File ~/micromamba/envs/awkward-docs/lib/python3.11/site-packages/awkward/_broadcasting.py:623, in apply_step.<locals>.broadcast_any_list()
620 nextinputs.append(x)
621 nextparameters.append(NO_PARAMETERS)
--> 623 outcontent = apply_step(
624 backend,
625 nextinputs,
626 action,
627 depth + 1,
628 copy.copy(depth_context),
629 lateral_context,
630 options,
631 )
632 assert isinstance(outcontent, tuple)
633 parameters = parameters_factory(nextparameters, len(outcontent))
File ~/micromamba/envs/awkward-docs/lib/python3.11/site-packages/awkward/_broadcasting.py:1086, in apply_step(backend, inputs, action, depth, depth_context, lateral_context, options)
1084 return result
1085 elif result is None:
-> 1086 return continuation()
1087 else:
1088 raise AssertionError(result)
File ~/micromamba/envs/awkward-docs/lib/python3.11/site-packages/awkward/_broadcasting.py:1055, in apply_step.<locals>.continuation()
1053 # Any non-string list-types?
1054 elif any(x.is_list and not is_string_like(x) for x in contents):
-> 1055 return broadcast_any_list()
1057 # Any RecordArrays?
1058 elif any(x.is_record for x in contents):
File ~/micromamba/envs/awkward-docs/lib/python3.11/site-packages/awkward/_broadcasting.py:672, in apply_step.<locals>.broadcast_any_list()
670 for x, x_is_string in zip(inputs, input_is_string):
671 if isinstance(x, listtypes) and not x_is_string:
--> 672 next_content = broadcast_to_offsets_avoiding_carry(x, offsets)
673 nextinputs.append(next_content)
674 nextparameters.append(x._parameters)
File ~/micromamba/envs/awkward-docs/lib/python3.11/site-packages/awkward/_broadcasting.py:372, in broadcast_to_offsets_avoiding_carry(list_content, offsets)
370 return list_content.content[:next_length]
371 else:
--> 372 return list_content._broadcast_tooffsets64(offsets).content
373 elif isinstance(list_content, ListArray):
374 # Is this list contiguous?
375 if index_nplike.array_equal(
376 list_content.starts.data[1:], list_content.stops.data[:-1]
377 ):
378 # Does this list match the offsets?
File ~/micromamba/envs/awkward-docs/lib/python3.11/site-packages/awkward/contents/listoffsetarray.py:411, in ListOffsetArray._broadcast_tooffsets64(self, offsets)
406 next_content = self._content[this_start:]
408 if index_nplike.known_data and not index_nplike.array_equal(
409 this_zero_offsets, offsets.data
410 ):
--> 411 raise ValueError("cannot broadcast nested list")
413 return ListOffsetArray(
414 offsets, next_content[: offsets[-1]], parameters=self._parameters
415 )
ValueError: cannot broadcast nested list
This error occurred while calling
numpy.multiply.__call__(
<Array [[], [21.9, ...], ..., [48.1, 38.7]] type='299973 * var * fl...'>
<Array [[-0.782, -0.997, -0.326], ..., []] type='299973 * var * flo...'>
)
This is data structure-aware, array-oriented programming.
Application: Filtering events with an array of booleans.
events.MET.pt, events.MET.pt > 20
(<Array [21.9, 17, 19.1, 30.9, ..., 17.7, 24, 12.9] type='299973 * float32'>,
<Array [True, False, False, True, ..., False, True, False] type='299973 * bool'>)
len(events), len(events[events.MET.pt > 20])
(299973, 163222)
Application: Filtering particles with an array of lists of booleans.
events.electron.pt, events.electron.pt > 30
(<Array [[], [21.9, ..., 23.8], ..., [48.1, 38.7]] type='299973 * var * float32'>,
<Array [[], [False, ..., False], ..., [True, True]] type='299973 * var * bool'>)
ak.num(events.electron), ak.num(events.electron[events.electron.pt > 30])
(<Array [0, 4, 2, 1, 4, 1, 1, 1, ..., 0, 3, 0, 1, 0, 2, 2] type='299973 * int64'>,
<Array [0, 2, 0, 0, 1, 0, 0, 0, ..., 0, 2, 0, 0, 0, 0, 2] type='299973 * int64'>)
Quizlet: Using the reducer ak.any()
, how would we select events in which any electron has $p_T > 30$ GeV/c$^2$?
events.electron[events.electron.pt > 30]
[[], [{pt: 42.6, eta: -0.98, phi: -1.86, mass: 0.00867, charge: 1, ...}, ...], [], [], [{pt: 30.7, eta: -0.61, phi: 1.01, mass: 0.0117, charge: -1, ...}], [], [], [], [{pt: 41, eta: -0.216, phi: 2.79, mass: -0.0128, charge: -1, ...}, {...}], [], ..., [], [{pt: 30.2, eta: 1.84, phi: 1.94, mass: -0.00882, charge: 1, ...}], [], [{pt: 37, eta: -2.35, phi: 0.903, mass: -0.0594, charge: 1, ...}, {...}], [], [], [], [], [{pt: 48.1, eta: 0.532, phi: -1.61, mass: 0.0127, charge: -1, ...}, ...]] --------------------------------------------------------------------------- type: 299973 * var * Momentum4D[ pt: float32, eta: float32, phi: float32, mass: float32, charge: int32, pfRelIso03_all: float32, dxy: float32, dxyErr: float32, dz: float32, dzErr: float32 ]
Awkward Array has two combinatorial primitives:
ak.cartesian()
takes a Cartesian product of lists from $N$ different arrays, producing an array of lists of $N$-tuples.
ak.combinations()
takes $N$ samples without replacement of lists from a single array, producing an array of lists of $N$-tuples.
numbers = ak.Array([[1, 2, 3], [], [4]])
letters = ak.Array([["a", "b"], ["c"], ["d", "e"]])
ak.cartesian([numbers, letters])
[[(1, 'a'), (1, 'b'), (2, 'a'), (2, 'b'), (3, 'a'), (3, 'b')], [], [(4, 'd'), (4, 'e')]] -------------------------------------------------------------- type: 3 * var * ( int64, string )
values = ak.Array([[1.1, 2.2, 3.3, 4.4], [], [5.5, 6.6]])
ak.combinations(values, 2)
[[(1.1, 2.2), (1.1, 3.3), (1.1, 4.4), (2.2, ...), (2.2, 4.4), (3.3, 4.4)], [], [(5.5, 6.6)]] -------------------------------------------------------------------------- type: 3 * var * ( float64, float64 )
Often, it’s useful to separate the separate the left-hand sides and right-hand sides of these pairs with ak.unzip()
, so they can be used in mathematical expressions.
electron_muon_pairs = ak.cartesian([events.electron, events.muon])
electron_muon_pairs.type.show()
299973 * var * (
Momentum4D[
pt: float32,
eta: float32,
phi: float32,
mass: float32,
charge: int32,
pfRelIso03_all: float32,
dxy: float32,
dxyErr: float32,
dz: float32,
dzErr: float32
],
Momentum4D[
pt: float32,
eta: float32,
phi: float32,
mass: float32,
charge: int32,
pfRelIso03_all: float32,
pfRelIso04_all: float32,
dxy: float32,
dxyErr: float32,
dz: float32,
dzErr: float32
]
)
electron_in_pair, muon_in_pair = ak.unzip(electron_muon_pairs)
electron_in_pair.type.show()
299973 * var * Momentum4D[
pt: float32,
eta: float32,
phi: float32,
mass: float32,
charge: int32,
pfRelIso03_all: float32,
dxy: float32,
dxyErr: float32,
dz: float32,
dzErr: float32
]
electron_in_pair.pt, muon_in_pair.pt
(<Array [[], [], [], [10.4, ...], ..., [], [], []] type='299973 * var * float32'>,
<Array [[], [], [], [54.3, ...], ..., [], [], []] type='299973 * var * float32'>)
ak.num(electron_in_pair), ak.num(muon_in_pair)
(<Array [0, 0, 0, 7, 0, 2, 1, 0, ..., 0, 0, 0, 2, 0, 0, 0] type='299973 * int64'>,
<Array [0, 0, 0, 7, 0, 2, 1, 0, ..., 0, 0, 0, 2, 0, 0, 0] type='299973 * int64'>)
To use Vector’s deltaR
method ($\Delta R = \sqrt{\Delta\phi^2 + \Delta\eta^2}$), we need to have the electrons and muons in separate arrays.
electron_in_pair, muon_in_pair = ak.unzip(ak.cartesian([events.electron, events.muon]))
electron_in_pair.deltaR(muon_in_pair)
[[], [], [], [1.07, 0.405, 1.97, 2.78, 1.01, 0.906, 2.79], [], [2.55, 0.8], [2.63], [], [], [], ..., [0.44, 3.16, 3.01, 1.05], [3.06, 1.52], [], [], [], [2.54, 0.912], [], [], []] ---------------------------------------------- type: 299973 * var * float32
first_electron_in_pair, second_electron_in_pair = ak.unzip(ak.combinations(events.electron, 2))
first_electron_in_pair.deltaR(second_electron_in_pair)
[[], [2.02, 2.35, 1, 0.347, 1.3, 1.64], [2.96], [], [3.31, 1.23, 2.39, 3.8, 3.19, 2.61], [], [], [], [1.18, 2.05, 2.98, 2.26, 2.59, 1.9], [], ..., [2.87], [], [], [2.05, 2.92, 3.02], [], [], [], [2.96], [2.72]] ------------------------------------- type: 299973 * var * float32
Quizlet: What’s this?
(first_electron_in_pair + second_electron_in_pair).mass
[[], [52.1, 76.8, 22.8, 19.9, 39.1, 64.2], [36], [], [87.3, 18.1, 28.5, 63.9, 56.5, 12.2], [], [], [], [19.8, 44.7, 92.2, 16.7, 38.7, 46.9], [], ..., [27.3], [], [], [39.2, 107, 38.4], [], [], [], [40.6], [91.5]] -------------------------------------- type: 299973 * var * float32
Hist.new.Reg(120, 0, 120, name="mass (GeV)").Double().fill(
ak.flatten((first_electron_in_pair + second_electron_in_pair).mass, axis=-1)
).plot();
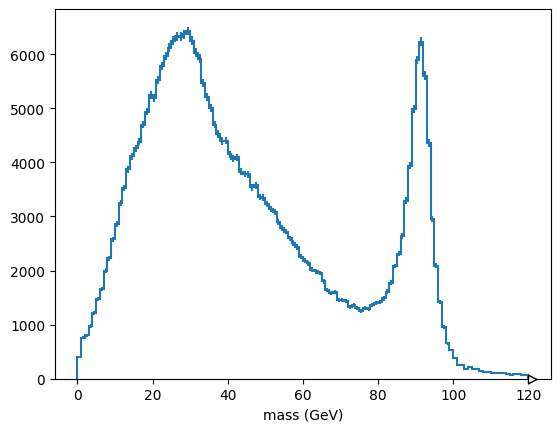